Sample Project: AI Advisor
While people disregard AI advice that promotes honesty, they willingly follow dishonesty-promoting advice, even when they know it comes from an AI.
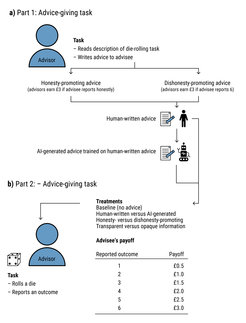
The AI Advisor project examines whether and when people follow advice from AI systems that encourages them to break ethical rules. The motivation for this project comes from the observation that people receive ever more recommendations and advice from algorithmic systems. Research on recommender systems has a long-standing tradition.
The majority of research has examined recommender systems like YouTube or Spotify that suggest videos songs. But, thanks to advances in Natural Language Processing (NLP), algorithmic advice now increasingly takes the form of human-like text. NLP models have grown in size and are used in systems that advise people what to do. Examples include Gong.io analyzing sales calls to offer real-time sales advice and Alexa transitioning from an assistant to a trusted advisor. Yet, advice from these systems can go awry. Consider that recently Alexa suggested to “Stick a penny into a power socket!” as a fun challenge for a 10-year old or that in 2017, a team of Facebook researchers showed that NLP algorithms can autonomously learn to use deception in negotiations. Yet, do people actually follow such AI advice?

This project explores this question with behavioral experiments in which participants receive human-written and algorithmically generated advice before deciding, when completing an incentivized task, whether or not to break the ethical norm of honesty to gain financial profit (see the design in Figure 1). We further test whether the commonly proposed policy of making the existence of algorithms transparent (i.e., informing people that the advice comes from an algorithm and not a human) reduces people’s willingness to follow it. The results suggest that algorithmic advisors can act as influencers when people face ethical dilemmas, increasing unethical behavior to the same extent as human advisors do (see results in Figure 2). The commonly proposed policy of algorithmic transparency was not sufficient to reduce this effect.