Open Science Innovation Award
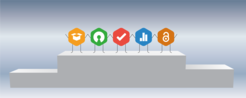
To promote awareness, foster exchange, and encourage collaboration in the realm of Open Science, the Institute has established an annual Open Science Innovation Award, starting in 2023.
The award will recognize and celebrate innovative ideas to enhance the accessibility, transparency, and reproducibility of the Institute’s research. Each year, two award winners (one individual and one team) will be invited to present their work in a designated event.
Here are this year's winner:
In this application for the Open Science Innovation Award, I want to present my involvement with the Brain Imaging Data Structure (BIDS).
BIDS is a very popular community-developed data standard for neuroimaging data.
However, while BIDS currently supports a vast array of neuroimaging modalities such as MRI, MEG, EEG, and NIRS,
the situation was different when I started my PhD in 2018:
EEG data was not a supported BIDS modality at that time, yet for my PhD I wanted to work with EEG data.
Back then, my supervisor encouraged me to look into BIDS and see whether it could be extended to support EEG data.
I thought that was a great idea, because data standards are indispensable as a foundation for good research data management.
Through such standards, we can make sure that reusability, reproducibility, and research efficiency are well enabled.
Luckily, I found a work group that had just started on preparing an inclusion of the EEG modality into BIDS.
I started contributing consistently in discussions surrounding the project, and was eventually invited as a co-lead of the project.
We added EEG as a modality for BIDS and it has been a very popular extension
(in the last 5 years, our paper describing the extension has been cited over 250 times).
Ever since, I have been heavily involved with BIDS and have overseen and facilitated the inclusion of many additional modalities into BIDS in my role as a lead maintainer of the project.
However, a data standard by itself can be cumbersome: Dataset curators have to read many pages of documentation and potentially write their own code to organize their data.
To support BIDS users with tools, colleagues and I have extended the popular EEG analysis software ""MNE-Python"" to work with BIDS.
Our result is a software called ""MNE-BIDS"". It is meant to simplify the conversion of EEG data to the BIDS format, and to read and analyze entire BIDS datasets.
We published a paper on MNE-BIDS in the Journal of Open Source Software (JOSS; over 60 times in the last 5 years).
At the institute I have given workshops for both BIDS (RDM workshop in 2022) and MNE-BIDS (MPDCC workshop in 2023).
All data that I collected during my PhD are organized in BIDS, well documented, and published openly.
Throughout my work on BIDS (including MNE-BIDS as a supporting software), I have learned several important lessons.
I want to close this application by listing three of these lessons below:
1) Support by my supervisors to work on these ""research adjacent"" projects was crucial. I wish that in the future, more and more supervisors provide their students with the opportunity to take time off their main research projects and invest in ""public good"" open science initiatives.
2) Working at ""open science junctions"" like the BIDS and MNE-Python projects provides you with opportunities to (i) network outside your own field, and (ii) become a part of a ""greater whole"" and to obtain a sense of concrete impact that is often lacking when working on smaller-scale, incremental basic research papers.
3) Sticking with the BIDS and MNE-BIDS projects as a maintainer has proven to be very valuable: The projects remain healthy and usable, and even keep growing. And at the same time, it is very gratifying to see people use these tools on a daily basis. I strongly advocate for applying the concept of ""maintaining your projects"" to all scientific projects, way beyond their commonly accepted ""end of life"" (which is all too often the date of the paper publication).
Relevant links:
Dissertation
Proof of being a BIDS maintainer
Proof of being on the MNE-Python steering council
BIDS paper on EEG extension
Other (recent) BIDS paper of relevance
Paper on MNE-BIDS software
Slides RDM workshop on BIDS
Proof MNE-Python workshop at MPDCC
Google Scholar profile with citation counts
Here are this year's submissions:
In the realm of the research project “The process structure of memory in early to middle childhood” I employed a range of Open Science practices to enhance the accessibility, reliability and reproducibility of my work.
The core manuscript of this project, which investigates memory development in a structural equation modelling framework, has been accepted as a Registered Report at stage 1 in Developmental Science and is available as a preprint on psyarXiv. This innovative publishing format not only addresses prevalent issues within the academic publishing system but also significantly elevates the quality and integrity of the proposed research. First, it secures the methodological soundness of the experimental design and planned analyses, as it allows to incorporate changes by reviewers before the start of data collection. Second, it increases transparency of all planned analyses, clearly demarking how reported results adhere to or deviate from pre-specified hypotheses. Third, it minimizes reporting and publication biases, as it bases the decision about publication on the quality of the proposed research, as opposed to the results found in the data.
Notably, the model comparisons entailed in this project make it challenging to derive estimates of statistical power via traditional analytical methods. Yet, lack of statistical power is a major cause of unreliable results in psychological research. In a related project, I therefore conducted an extensive Monte-Carlo simulation-based power analysis for the comparison of non-nested structural equation models, to ensure an adequate sample size for the research questions at hand. This kind of power simulation is not trivial, particularly for researchers with limited expertise in Monte-Carlo simulations or structural equation modelling. To make the application of this approach accessible to a broader audience, I expanded this analysis into a tutorial paper, which provides a step-by-step guide for researchers unfamiliar with this approach. This tutorial assumes very little prior knowledge, and therefore aims at empowering researchers with limited methodological expertise to conduct well-powered analyses in their own research endeavors. This tutorial paper is currently under review for publication at Behavioral Research Methods, and already accessible as a preprint on github along with fully open, version controlled code. The manuscript is further dynamically generated as an RMarkdown file to ensure reproducibility of the report and increase the transparency of the reported results.
By increasing the transparency and accessibility of my work from conceptualization to analysis and reporting, I hope to minimize ambiguities in the research process, which can significantly harm scientific gain. I further believe that a key asset of Open Science practices lies in fostering a truly cumulative science, in which findings, datasets, and tools can be re-used by other scientists.
While the employment of Open Science practices, such as the ones described above, undoubtedly enhance the quality, transparency, and scientific value of the work, they also entail notable additional time and effort. Particularly for researchers at an early career stage, this additional strain can pose challenges within a restricted timeline of publishing scientific articles for a dissertation. Despite increasing awareness in the scientific community regarding the importance of Open Science practices, contract conditions and evaluation criteria for PhD theses often do not fully reflect this additional challenge. In the case of my dissertation, receiving support from my supervisor, second reviewer, and co-authors to include a stage 1 registered report as part of my dissertation was invaluable. This underscores the importance of mentorship and institutional support in facilitating the embrace of Open Science practices by junior researchers.
Relevant links:
The process structure of memory abilities in early and middle childhood, Stage 1 registered report
https://osf.io/preprints/psyarxiv/p84a6
Estimating Statistical Power for Structural Equation Models in Developmental Cognitive Science: A Tutorial in R
https://link.springer.com/article/10.3758/s13428-024-02396-2
https://github.com/ebuchberger/Estimating-Power-for-SEM
Further team members:
Jane Rebecca Conway
Jean-François Bonnefon
Azim Shariff
Iyad Rahwan
It is a cross-cultural study, in which we proposed and empirically tested a psychological model to explain the global variations of people’s fear of AI across 20 different countries. Despite various challenges, we followed the best practices of Open Science across different stages of the project, including detailed preregistrations, open data, and open materials.
At the very beginning, all our team members already agreed to test clearly defined research questions and confirmatory hypotheses, avoiding post-hoc justifications. However, the novel and multi-nation nature of the study made it challenging to formulate a hypothesis with supporting literature. Therefore, we ran a pilot study with a convenient sample. Based on this pilot data, we articulated our hypothesis with more confidence, developed the analysis codes for the main study, and justified statistical power for the intended sample size (total N = 10,000). We then preregistered the main study on Open Science Framework [https://tinyurl.com/cultureAIfearPreregister], providing information about our hypothesis, design and materials, sampling plan and power analysis, exclusion criteria, and analysis plan with actional analysis codes.
We then performed the main study and analyzed the data. At this stage, we increasingly realized that the investments in preregistration paid off and made follow-up steps efficient. The preregistered documents provided clear guidelines for team-coordinated data collection and analysis, which were extremely helpful given the large volume and complex structure of our data. Also, the preregistered analysis plan did not constrain us from exploring the data from other interesting perspectives. Instead, it reminded us of the distinction between our confirmatory and exploratory analyses, which turned into different sections in our research report. Such a distinction can send clearer signals to readers about the well-grounded takeaways (based on our confirmatory analyses) and promising future directions (based on our exploratory analyses).
After writing up the research report, we believed that a timely dissemination of our scientific findings (about how and why people from different countries are afraid of AI in sensitive occupations) can be crucial to many scholars and practitioners. Therefore, we published the working paper on the preprint repository PsyArXiv [https://osf.io/preprints/psyarxiv/pjvqt]. For those interested in recycling our materials, reproducing our results, or exploring our valuable large-scale multi-nation data, we made our study materials, data, and analysis codes available [https://tinyurl.com/cultureAIfear]. The preprint page also presents links to access these technical details while reading the paper.
Completing this study was a rewarding experience, but there is still much to learn. For example, many journals are promoting a new publishing format called Registered Report, which allows researchers to submit study proposals and receive in-principle acceptance (IPA) before commencing data collection. This new format can help reduce reporting and publication biases. Although we tried this approach earlier, hoping to improve our experiment design with reviewers’ feedback before the costly data collection, we faced challenges conveying the merits of our proposed study without the actual rich data from different countries.
Now, the study is completed and under review in a psychology journal. We received positive feedback from reviewers on our Open Science practices. We also experienced another advantage of Open Science practices at the peer-review stage: Although our proposed model runs counter to some well-established theories tested mainly in one or a few Western countries, Open Science practices help present our findings as well-justified and consolidate our arguments.
We hope everyone can learn and benefit from Open Science practices in their research, as we did here and will continue in our future work.
Relevant links:
Preregistration
Materials, data, and analysis codes
Preprint
Further team members:
Dr. Marit Petzka
Our study “The Effect of Sleep on Insight” is a pre-registered conceptual replication of Lacaux et al. (2021) who found a benefit of particularly N1-sleep on insight. Conceptual replication is a key component of the scientific process contributing to a more robust understanding and improved reliability. It increases the validity, as well as the generalizability of findings by testing the phenomena under different settings and conditions. To this end, we used the same experimental protocol as Lacaux et al.(2021), but tested the hypotheses with our own behavioural insight task (Löwe et al., 2023). Specifically, participants completed 4 task blocks before being given a 20-minute rest period to nap during which EEG was recorded. After the rest, participants completed 5 more blocks during which insight was measured. Based on the findings of the original paper, we tested the following hypotheses:
1) N1-sleep compared to wakefulness leads to a higher number of insight about a hidden strategy.
2) N2-sleep compared to wakefulness leads to a reduced number of insight about a hidden strategy.
Pre-registrations are critical for promoting transparency and reducing bias while increasing rigour and reliability of research. They render the scientific process more trustworthy since hypotheses and analyses have to be described in detail before data is acquired. Accordingly, deviations from the registered analysis approach have to be clearly motivated and flagged. This, in consequence, helps combat p-hacking and HARKing (Hypothesizing After the Results are Known). Further, pre-registering improves the quality of research by encouraging researchers to carefully plan and motivate design choices such as sample size and methodological details.
In line with these practices, we chose the Bayesian updating method (Moerbeek, 2021) for our study to determine the appropriate sample size to draw meaningful conclusions. This method allows researchers to increase the sample size to obtain sufficient evidence for or against the hypotheses. Specifically, we determined a minimum sample size of 50 and maximum of 100 participants (the latter being defined based on Lacaux et al. (2021)). Three scenarios were defined as stopping criteria: 1) evidence (BF10 >= 6) for the alternative hypothesis is present, 2) evidence for the null hypothesis is present (BF01 >= 6) or 3) data of 100 participants have been collected.
We pre-registered an exclusion criterion based on task performance in the last block of the pre-nap part. Participants whose performance on the easiest trial levels was below 80% were excluded. At 67 included subjects, we find no evidence for Hypothesis 1 (BF10 = 0.883), but for the null hypothesis (BF01 = 8.161). Upon applying the pre-registered stopping rule for Hypothesis 2, we noticed an error in our formulation of the hypothesis. Based on the effects of the original Lacaux et al. paper, we should have registered the hypothesis that N2-sleep leads to reduced insight compared to N1-sleep, not wakefulness. For paper submission, we will handle this error according to suggestions outlined by Lakens (2024). For the corrected hypothesis, we observe strong evidence (BF01 = 63.675) against (for the erroneously pre-registered hypothesis: BF01 = 8.17). Our results show that insight depends on sleep (Fisher’s exact test, p = 0.042). However, contrary to the N1-sleep effect reported in the original paper, people who had N2-sleep were more likely to have insight. Only for the N2-sleep group (83%), but neither for the N1-sleep (54%) nor wake rest group (53%) does the insight ratio differ from the insight baseline derived from our previous work (50%) (Löwe et al., 2023). Our study not only shows how open and transparent research can be conducted (pre-registration; handling errors in pre-registrations) but also how important (conceptual) replications are to scientifically progress.
Relevant links:
OSF Project Page
Science Advances Article
arXiv Preprint
Further team members:
Dorothee Mischkowski
Zoe Rahwan
Christoph Engel
1) Introduction:
We apply as a team with an in-principle accepted Registered Report at a fully open-access journal from the Nature portfolio to the MPIB Open Science Innovation Award. Our Stage 1 Registered Report is openly available as a preregistration at Figshare, and we have made a preprint of our Stage 2 Registered Report available under an open license (CC-BY 4.0) at the Open Science Framework. Our preprint has also been made openly available at MPG.PuRe, SSRN, EconPapers – RePeC, and in an MPI Discussion Paper Series. We have made all of our power analyses, study materials, anonymized data sets, and analysis pipelines openly available for both of our pilot studies and our main study. Our Registered Report has been accepted in principle under a transparent peer review system: Editorial decision letters, including reviewers' comments and the authors’ rebuttal letters are published online as a supplementary peer review file. We have employed rigorous, open-access measures in our Registered Report to support reproducibility and uphold best practices in open science.
2) Motivation:
We have used open practices to make our research reproducible, robust, and reliable. More specifically, we had three reasons for using open research practices in our Registered Report. First, the Registered Report format allowed us to increase the methodological rigor of our work. By only collecting data after the peer review process, we could incorporate feedback effectively at the time where it was most helpful. In particular, the Registered Report feedback helped us strengthen our study design when we could still make important methodological adjustments. For example, feedback from both editors and reviewers helped us to base our effect size estimates on more solid footing. This strengthened our power analysis, increasing the rigor in our sample size calculations, which we made openly available during our Stage 1 review process. If we had entered the peer review process after collecting data, this would not have been possible. Second, the Registered Report format allowed us to counteract own biases. An upload of our analysis pipeline together with pilot data to the Open Science Framework clearly specified which confirmatory tests we would run, and which tests we did not plan. By committing ourselves to clearly defined hypotheses and a confirmatory analysis plan, we minimized wiggle room, while still leaving space for exploratory analyses. We included these in our Stage 2 manuscript, which is currently under Stage 2 review. Finally, we chose the Registered Report format to counteract publication bias. Given prominent constraints to the reproducibility of psychological science (Open Science Collaboration, 2015), we wanted to use a format that focused more on the importance of our research questions and the rigor of our methods than on the results of our study. We view compliance with open science principles as positive and rewarding. This Registered Report is one of four that has been accepted or accepted in principle within our team. We see Registered Reports as an important Open Science Innovation, while continuously learning from our experiences with the format.
3) Lessons learned:
Pilot data is often listed as an optional element for Registered Reports. Yet, when a research design is novel, it can be crucial to demonstrate feasibility. We had initially submitted our Stage 1 Registered Report without any pilot data. Based on the editors’ and reviewers’ feedback, we collected pilot data to demonstrate feasibility. We have learned from this process to carefully consider which design elements are novel, and, if in doubt, already submit pilot data as part of the Stage 1 submission. This will support the Registered Report review process and, ultimately, contribute to the success of the open science project.
Relevant links:
Registered Report (Stage 1 Manuscript)
OSF Page with Study Materials, Data, Code, and Preprint (CC-BY 4.0)
MPI Landing Page for Preprint
MPI Discussion Paper Series
MPI Series on SSRN
MPI Series on EconPapers / RePec
MPS Repository
In the master's thesis titled "In-Depth Hyperparameter Selection For Layer-Wise Relevance Propagation", the following Open Science practices were implemented to enhance transparency and reproducibility, addressing a recurring issue in scientific research.
Open Source: The complete source code used in the research was published on GitHub, allowing other researchers and practitioners to review, use, and modify it.
Open Access Manuscript: The full manuscript of the thesis was made accessible online, ensuring that the research findings and methodologies are available to a broader audience beyond those with institutional access to academic journals.
Open Data: The data used is openly accessible to ensure the reproducibility of results.
Open License: The code is available under the MIT License, while the manuscript is available under the Creative Commons Attribution 4.0 International License. These licenses allow others to use and build upon the work as long as the original copyright notice is included.
Open-Source Software Package: An open-source Python package was published on the Python Package Index (PyPI) for the reusability and reproducibility of the research findings. This package is version-controlled, highlighting the evolution of the project and maintaining transparency in the development process.
The thesis addressed the issue of interpretability in machine learning (ML), with a particular focus on the algorithm Layer-Wise Relevance Propagation (LRP), a technique for understanding the outputs of neural networks. The core of the research involved a quantitative and qualitative evaluation of LRP rules, using Pixel-Flipping. This approach demonstrated that the choice of evaluation metrics and heatmap visualization techniques can significantly impact the interpretability of neural networks. The findings emphasized the significance of context and subjectivity in visual explanations within ML, suggesting that the requirements for interpretability should be defined on a case-by-case basis.
The adoption of Open Science practices in this research was primarily motivated by the desire to ensure reproducibility and accessibility. This approach was informed by observations in the field, where a pervasive lack of transparency and reproducibility in existing research was often a significant barrier to validating its findings. Open Science principles address these challenges and contribute to advancing a more open and collaborative scientific community.
The impact of these Open Science practices extends beyond the immediate scope of this thesis. For instance, the open-source package has been downloaded roughly seven thousand times, demonstrating its practical utility and the value of making scientific tools readily available.
The process of meticulously documenting the code to enhance user-friendliness, while demanding in terms of effort, was critical in guaranteeing the research's reproducibility. Additionally, publishing a software package required meticulous documentation of the meta-data for accessibility and adaptation of the code. Professional experience in Software Engineering proved instrumental in surmounting these challenges.
Relevant links:
Open access manuscript
Open source code and data
Open-source software package
This contribution underscores an innovative venture at our institution, utilizing functional Near-Infrared Spectroscopy (fNIRS) in a scientific landscape that progressively focuses on complex data acquisitions. Under Prof. Simone Kühn’s guidance since early 2023, I initiated fNIRS project on neurocognitive dynamics of summetry processing within working memory. Given the novelty of NIRS in our open-access environment —contrasted with established protocols of EEG and MRI—my objective was foundational, aiming for transparency and reproducibility in our experimental approach, thereby setting precedent for subsequent studies. This effort entailed the pre-registration of research design, open sharing of experiment code, data, and analysis procedures (via OSF), and prompt dissemination through preprint and submissions to open-access journals, such as Scientific Reports. Moreover, all device-handling notes and manuals serve training purposes for future fNIRS users within the MPIB. My approach pioneers methodological framework incorporating Open Science by default within our institute and aspires to uplift standards across the fNIRS community, traditionally less engaged with Open Science principles compared to EEG or fMRI fields.
The motivation behind integrating Open Science practices into this research was twofold. Firstly, I aimed to enhance the credibility and reproducibility of my findings. The research conducted in this project focused on symmetry's ubiquity in the visual environment and its underexplored role in working memory. Given limited understanding of symmetry’s role in cognition and novel nature of the experimental paradigm, offering comprehensive insights through openly shared resources had many benefits. By openly sharing the methodology, data, and findings, I aimed to provide transparency and early insights, as well as open the results to a debate on their potential implications. By making our research design, data, and analysis processes publicly accessible, I also invite scrutiny, validation, and further exploration of my work, fostering trust in scientific process and collaborative advancement in neuroscience. Secondly, my approach was intended to democratize access to neuroscientific knowledge and resources. By sharing the project openly, I hope to encourage researchers within our institute to try the fNIRS method to cultivate use of the resource.
This project has yielded several insightful lessons worth highlighting. First of all, there was poor community support when the project began. As mentioned, fNIRS community is relatively small and is still trying to figure out how to implement Open Science practices best. The institute, as relatively new to the field, is not embedded within this community enough to have the support of researchers. That obstacle translated into our institutional uncertainties regarding the standards we should apply regarding data and code sharing. Thankfully, our RDM team is knowledgeable, and we were able to extrapolate the most relevant rules (like BIDS formatting) from other methods (EEG or fMRI). Much skepticism regarding the fNIRS is based on poor reporting methods and difficulties in validating the outcomes. Preregistrations are rare, and openly available datasets are scarce. That builds mistrust that is visible not only in the broad neuroscientific community but also in our institute. I endeavored to show our community how reliable and efficient the method can be if the research pipeline is transparently accessible to everyone.
By pioneering these practices in our fNIRS research, I hoped to contribute to the field's methodological rigor and advocate for a shift toward greater transparency and collaboration. My work thus benefits the broader scientific community, setting a precedent for future research and encouraging a systemic shift towards more open and reproducible science.
Relevant links:
Preregistration
Project's OSF repository
Preprint