Sample Project: Lie Detection
Lie detection algorithms attract few users but vastly increase accusation rates.

The study consisted of two parts. In Part 1, participants (=authors) wrote one true and one false statement; in Part 2, a separate sample of participants (=judges) judged four statements. Four different treatments existed. In the baseline treatment, participants decided by themselves, without fact-checking (=all accusations led to a reduction of payoffs for the author); in the fact-checking treatment, participants decided by themselves, with fact-checking (only accusations that were justified led to a reduction of payoffs for the author); in the lie-detection algorithm treatment, judges could purchase the advice from a lie-detection algorithm, but fact-checking was not present; in the both treatment, judges could purchase advice from a lie-detection algorithm.
People are not very good at detecting lies, which may explain why they refrain from accusing others of lying, given the social costs attached to false accusations—both for the accuser and the accused. In the Algorithmic Lie Detection project, we consider how this social balance might be disrupted by the availability of lie-detection algorithms powered by Artificial Intelligence. Will people elect to use lie detection algorithms that perform better than humans and, if so, will they show less restraint in their accusations? We built a machine learning classifier whose accuracy (67%) was significantly better than human accuracy (50%) in a lie-detection task, and conducted an incentivized lie-detection experiment in which we measured participants’ propensity to use the algorithm, as well as the impact of that use on accusation rates (for an overview of the study design, see Figure 1).
Our results reveal that only a few people (33%) who elect to use the algorithm drastically increase their accusation rates (from 25% in the baseline condition up to 86% when the algorithm flags a statement as a lie). They make more false accusations (18pp increase), while the probability of a lie remaining undetected is much lower in this group (36pp decrease). We consider individual motivations for using lie detection algorithms and the social implications of these algorithms (see Figure 2 for results).
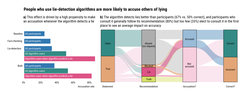
(A) Accusation rate in each of the four treatments. In the lie-detection algorithm treatment and the both treatment (=where participants had access to our AI lie detection algorithm), accusation rates are also shown for the subset who elected to use the algorithm, as well as for the sub-subset of these participants whose lie-detection algorithm tagged the target statement as a lie. (B) Detailed stage-by-stage data for the lie-detection algorithm treatment and the both treatment, showing the AI’s guess (not requested, blocked, lie, truth) for false and true target statements, the subsequent decision of the participant depending on the algorithm’s guess, and the accuracy of this decision.
Scientific writings
von Schenk, A., Klockmann, V., Bonnefon, J. F., Rahwan, I., & Köbis, N. (2022). Lie detection algorithms attract few users but vastly increase accusation rates. arXiv preprint arXiv:2212.04277.