Core Research Fields
These are the research fields that the Lifespan Neural Dynamics Group (LNDG) focuses on in its quest to understand how and why the human brain fluctuates so markedly from moment to moment.
Lifespan Development
Degradation of Neural Dynamics with Age
Early theory on aging, and in particular, the age-based degradation of cognition, historically ascribes decline to some form of heightened “neural noise” that interferes with efficient processing (e.g., Crossman & Szafran, 1956; Welford, 1965). However, these early theories had rarely been tested by examining within-subject brain signals directly. Perhaps counterintuitively, our extant in vivo work consistently shows that older, poorer performers may generally exhibit less moment-to-moment brain signal variability than younger, higher performing adults (e.g., Garrett et al., 2011, 2015; Garrett, Samanez-Larkin et al., 2013; Waschke, Kloosterman et al., 2021). Overall, our group seeks to understand why healthy, higher performing brains are highly and robustly dynamic across moments (adaptability, dynamic range, multi-stability?), and how this changes with development and aging.
Cognition
Behavioral Relevance of Neural Dynamics
Our interests in the relations between cognition and brain signal variability have been broad to date, spanning simple and complex perceptual decision-making (e.g., Kloosterman et al., 2019, 2020), internal vs. externally modulated states (e.g., Grady & Garrett, 2018), working (e.g., Garrett et al., 2015) and long-term memory (Waschke et al., 2023, bioRxiv), and reinforcement learning using a variety of stimuli types, cognitive performance metrics, and model parameterizations. Broadly, we find that better performers have higher brain signal variability, and that within-person signal variability can parametrically tune with changes in task difficulty (Garrett et al., 2014, 2015; Garrett, Kovacevic et al., 2013). We also continue to examine whether variability may be an expression of the flexible modulation of brain states that allows a probabilistic search for an optimal state, given either unexpected or anticipated changes in the environment (see Garrett, Samanez-Larkin et al., 2013; Waschke, Kloosterman et al., 2021).
Structural/Functional Connectivity
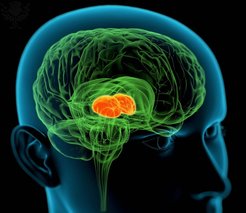
A theoretically and computationally informed contributing factor to brain signal variability effects is that our neural system can explore a broad repertoire of brain states/networks from moment to moment when variability is present (Deco et al., 2009, 2011; Garrett, Samanez-Larkin et al., 2013). Accordingly, a healthy, developed brain (i.e., in young adults) may be more flexible in the face of changing systemic or environmental demands, as it can reconfigure efficiently as required. Assuming that node-based signal variability reflects a dynamic system that is constantly reconfiguring in strength and direction, it is clear that explicitly measuring network-level dynamics is required to determine the bounds of node-based dynamics. We are examining these issues in detail within the LNDG. Our recent attempts to link node-wise temporal dynamics to network-level effects (e.g., Garrett et al., 2018) revealed that the vast majority of local temporal variability may be a reflection of network communication/integration rather than local “noise.” At the same time, single nodes can set off a dynamic cascade of activity across entire networks, revealing that single nodes remain essential for understanding network activity overall (Krohn et al., 2023). Crucially, the thalamus provided a unique and fundamental variability-based signature of how the brain integrates across scales, providing a key anatomical target for future LNDG work probing the generation and mechanisms of temporal variability in the brain at rest and on task (e.g., Garrett et al., 2022, bioRxiv). This perspective was profiled in our recent piece on the future of thalamic neuroimaging in Nature Reviews Neuroscience (Shine et al., 2023).
Neuromodulation
Neurochemical Bases for Neural Variability
We are interested in linking theories of dopamine (DA)-based neural “precision” (e.g., Ballo et al., 2012; Coull et al., 2012; Friston, 2010) and dynamics to brain signal variability effects. It is an interesting paradox that signal variability often appears functional for healthy neural systems, yet optimal DA function is presumed to generally invoke tighter bounds on temporal coding. We are currently collaborating with various research groups in Europe to investigate how DA agonists, antagonists, and PET-based DA binding potential may relate to working memory- and reinforcement-learning-based brain signal variability in young and older adults. We find that DA agonism increases BOLD variability in older adults (Garrett et al., 2015), DA antagonism decreases BOLD variability in younger adults (Garrett et al., in prep.), and DA binding (using PET imaging) correlates with BOLD variability in a regionally-dependent manner (Guitart-Masip et al., 2016; Garrett et al., 2022, bioRxiv). DA thus continues to be a primary neurochemical target within the LNDG, with our interests recently expanding to include the norepinephrine (e.g., as a modulator of uncertainty; Kosciessa et al., 2021) and glutamate/GABA systems (e.g., state-configuration via excitation/inhibition; Lalwani et al., 2021; Lalwani et al., 2022, bioRxiv).
Transcranial Stimulation
Can We Boost Deficient Dynamics?
Our initial studies in this domain focus on directly invoking stochastic resonance, which is classically observed when the presence of additive noise allows input signals to be better detected in the brain. Historically, “noise” has been experimentally applied to the nervous system in a number of ways via noisy peripheral visual/tactile/auditory stimuli (e.g., Collins et al., 1996; Wells et al., 2005). However, good evidence for whether/how these peripherally applied “noise” sources reach the brain has been lacking. Alternatively, transcranial noise stimulation (tRNS) methods provide a more direct route to the cortex of the brain, thus piquing our interest in simultaneous tRNS-neuroimaging to examine links between stimulation and brain signal dynamics. Overall, we seek to use tRNS as a “causal” technique for restoring impoverished signal variability levels in older adults.
Methods
Measures
We continue to both develop and apply brain signal variability methods, moving beyond general variance measures to ask more explicit questions about the nature of that variance (e.g., spectral content and structure, time delay embedding). We are also inherently interested in discrete patterns in brain signals (e.g., entropy), and how they relate to overall variance (Grandy et al., 2016). For example, we continue to optimize the estimation of multi-scale entropy that effectively eliminates variance-based biases from computation (Kloosterman et al., 2020; Kosciessa, Kloosterman et al., 2020), permitting entropy to be directly compared with oscillatory dynamics within-person (e.g., Kosciessa, Grandy et al., 2020; Kosciessa, Kloosterman et al., 2020).
Statistical and Computational Modelling
We have a keen interest in multivariate models (e.g., singular value decomposition (SVD)-based techniques) that best enable various correlates of signal dynamics to be simultaneously examined. We are currently exploring how SVD models can be optimized within a mixed-modeling framework in an attempt to link latent-level parametric cognitive performance to parametric task-based brain dynamics (e.g., Garrett, Kovacevic et al., 2013; Garrett et al., 2015). We also continue to develop whole-to-whole brain multivariate models linking different neuroimaging modalities (e.g., ASL and BOLD; dopamine PET and BOLD; EEG and BOLD; DWI and BOLD; e.g., Garrett et al., 2017; Burzynska et al., 2013).
We also utilize and develop various computational models of brain and behavior. For example, we have recently leveraged the HMAX model of the ventral visual cortex to estimate how the complexity of visual input may drive neural variability during perception (Garrett et al., 2020; Waschke et al., 2022, bioRxiv). With regard to behavior, we have also modelled within-person, reward-driven perceptual decision-making via hierarchical drift diffusion modelling (e.g., Garrett et al., 2022, bioRxiv; Kloosterman et al., 2019; Kosciessa et al., 2021), and now deploy various reinforcement-learning models across a host of probabilistic reward-based paradigms.
Software Development
An important goal of our proposed work is to support the future examination of brain signal variability in neuroimaging through open-source software. At present, no analysis package provides for a comprehensive brain signal variability analysis. As such, we continue to develop an event-related and block-design fMRI-based Variability Toolbox (VarTbx) for SPM. Mimicking a Level-1 analysis, our toolbox allows SPM users (or more generally, Matlab users) to import any preprocessed fMRI data, choose from a number of different variability measures, and to output resulting individual maps for further analysis within SPM’s standard Level-2 module (or elsewhere). The first version of VarTbx is now available; see our software page for details. We further support open science by making all code/algorithms freely available on our website and LNDG Github repository.
References
Ballo, A. W., Nadim, F., & Bucher, D. (2012). Dopamine modulation of Ih improves temporal fidelity of spike propagation in an unmyelinated axon. Journal of Neuroscience, 32(15), 5106–5119. https://doi.org/10.1523/JNEUROSCI.6320-11.2012
Burzynska, A. Z., Garrett, D. D., Preuschhof, C., Nagel, I. E., Li, S.-C., Bäckmann, L., Heekeren, H. R., & Lindenberger, U. (2013). A scaffold for efficiency in the human brain. Journal of Neuroscience, 33, 17150–17159. https://doi.org/10.1523/jneurosci.1426-13.2013
Collins J. J., Imhoff T. T., & Grigg P. (1996). Noise-enhanced tactile sensation. Nature, 383, 770. https://doi.org/10.1038/383770a0
Coull, J. T., Hwang, H. J., Leyton, M., & Dagher, A. (2012). Dopamine precursor depletion impairs timing in healthy volunteers by attenuating activity in putamen and supplementary motor area. Journal of Neuroscience, 32(47), 16704-16715. https://doi.org/10.1523/JNEUROSCI.1258-12.2012
Crossman, E. R., & Szafran, J. (1956). Changes with age in the speed of information-intake and discrimination. Experientia, Suppl. 4, 128–135.
Deco, G., Jirsa, V. & McIntosh, A. (2011). Emerging concepts for the dynamical organization of resting-state activity in the brain. Nature Reviews Neuroscience, 12, 43–56. https://doi.org/10.1038/nrn2961
Deco, G., Jirsa, V., McIntosh, A. R., Sporns, O., & Kötter, R. (2009). Key role of coupling, delay, and noise in resting brain fluctuations. Proceedings of the National Academy of Sciences of the United States of America, 106(25), 10302–10307. https://doi.org/10.1073/pnas.0901831106
Friston, K. (2010). The free-energy principle: A unified brain theory? Nature Reviews Neuroscience, 11, 127–138. https://doi.org/10.1038/nrn2787
Garrett, D. D., Epp, S. M., Kleemeyer, M., Lindenberger, U., & Polk, T. A. (2020). Higher performers upregulate brain signal variability in response to more feature-rich visual input. NeuroImage, 217, Article 116836. https://doi.org/10.1016/j.neuroimage.2020.116836
Garrett, D. D., Epp, S. M., Perry, A., & Lindenberger, U. (2018). Local temporal variability reflects functional integration in the human brain. NeuroImage, 183, 776–787. https://doi.org/10.1016/j.neuroimage.2018.08.019
Garrett, D. D., Kloosterman, N. A., Epp, S., Chopurian, V., Kosciessa, J. Q., Waschke, L., Skowron, A., Shine, J. M., Perry, A., Salami, A., Rieckmann, A., Papenberg, G., Wåhlin, A., Karalija, N., Andersson, M., Riklund, K., Lövdén, M., Bäckman, L., Nyberg, L., & Lindenberger, U. (2022, May 5). Dynamic regulation of neural variability during working memory reflects dopamine, functional integration, and decision-making. BioRxiv. https://doi.org/10.1101/2022.05.05.490687
Garrett, D. D., Kovacevic, N., McIntosh, A. R., & Grady, C. L. (2011). The importance of being variable. Journal of Neuroscience, 31(12), 4496–4503. https://doi.org/10.1523/JNEUROSCI.5641-10.2011
Garrett, D. D., Kovacevic, N., McIntosh, A. R., & Grady, C. L. (2013). The modulation of BOLD variability between cognitive states varies by age and processing speed. Cerebral Cortex, 23, 684–693. https://doi.org/10.1093/cercor/bhs055
Garrett, D. D., Lindenberger, U., Hoge, R., & Gauthier, C. J. (2017). Age differences in brain signal variability are robust to multiple vascular controls. Scientific Reports, 7, Article 10149. https://doi.org/10.1038/s41598-017-09752-7
Garrett, D. D., McIntosh, A. R., & Grady, C. L. (2014). Brain signal variability is parametrically modifiable. Cerebral Cortex, 24, 2931–2940. https://doi.org/10.1093/cercor/bht150
Garrett, D. D., Nagel, I. E., Preuschhof, C., Burzynska, A. Z., Marchner, J., Wiegert, S., Jungehülsing, G., Nyberg, L., Villringer, A., Li, S.-C., Heekeren, H. R., Bäckman, L., & Lindenberger, U. (2015). Amphetamine modulates brain signal variability and working memory in younger and older adults. Proceedings of the National Academy of Sciences of the United States of America, 112, 7593–7598. https://doi.org/10.1073/pnas.1504090112
Garrett, D. D., Samanez-Larkin, G. R., MacDonald, S. W. S., Lindenberger, U., McIntosh, A. R., & Grady, C. L. (2013). Moment-to-moment brain signal variability: A next frontier in human brain mapping? Neuroscience & Biobehavioral Reviews, 37(4), 610–624. https://doi.org/10.1016/j.neubiorev.2013.02.015
Grady, C. L., & Garrett, D. D. (2018). Brain signal variability is modulated as a function of internal and external demand in younger and older adults. NeuroImage, 169, 510–523. https://doi.org/10.1016/j.neuroimage.2017.12.031
Grandy, T. H., Garrett, D. D., Schmiedek, F., & Werkle-Bergner, M. (2016). On the estimation of brain signal entropy from sparse neuroimaging data. Scientific Reports, 6, Article 23073. https://doi.org/10.1038/srep23073
Guitart-Masip, M., Salami, A., Garrett, D., Rieckmann, A., Lindenberger, U., & Bäckman, L. (2016). BOLD variability is related to dopaminergic neurotransmission and cognitive aging. Cerebral Cortex, 26, 2074–2083. https://doi.org/10.1093/cercor/bhv029
Kloosterman, N. A., de Gee, J. W., Werkle-Bergner, M., Lindenberger, U., Garrett, D. D., & Fahrenfort, J. J. (2019). Humans strategically shift decision bias by flexibly adjusting sensory evidence accumulation. eLife, 8, Article e37321. https://doi.org/10.7554/eLife.37321
Kloosterman, N. A., Kosciessa, J. Q., Lindenberger, U., Fahrenfort, J. J., & Garrett, D. D. (2020). Boosts in brain signal variability track liberal shifts in decision bias. eLife, 9, Article e54201. https://doi.org/10.7554/eLife.54201
Kosciessa, J. Q., Grandy, T. H., Garrett, D. D., & Werkle-Bergner, M. (2020). Single-trial characterization of neural rhythms: Potential and challenges. NeuroImage, 206, Article 116331. https://doi.org/10.1016/j.neuroimage.2019.116331
Kosciessa, J. Q., Kloosterman, N. A., & Garrett, D. D. (2020). Standard multiscale entropy reflects neural dynamics at mismatched temporal scales: What's signal irregularity got to do with it? PLoS Computational Biology, 16(5), Article e1007885. https://doi.org/10.1371/journal.pcbi.1007885
Kosciessa, J. Q., Lindenberger, U., & Garrett, D. D. (2021). Thalamocortical excitability adjustments guide human perception under uncertainty. Nature Communications, 12, Article 2430. https://doi.org/10.1038/s41467-021-22511-7
Krohn, S., von Schwanenflug, N., Waschke, L., Romanello, A., Gell, M., Garrett, D. D., & Finke, C. (2023). A spatiotemporal complexity architecture of human brain activity. Science Advances, 9(5), Article eabq385. https//doi.org/10.1126/sciadv.abq3851
Lalwani, P., Garrett, D. D., & Polk, T. A. (2021). Dynamic recovery: GABA agonism restores neural variability in older, poorer performing adults. Journal of Neuroscience, 41(45), 9350–9360. https://doi.org/10.1523/JNEUROSCI.0335-21.2021
Lalwani, P., Polk, T. A., & Garrett, D. D. (2022, September 17). Modulation of neural variability: Age-related reduction, GABAergic basis, and behavioral implications. BioRxiv. https://doi.org/10.1101/2022.09.14.507785
Shine, J. M., Lewis, L. D., Garrett, D. D., & Hwang, K. (2023). The impact of the human thalamus on brain-wide information processing. Nature Reviews Neuroscience, 24, 416–430. https://doi.org/10.1038/s41583-023-00701-0
Waschke, L., Kamp, F., van den Elzen, E., Krishna, S., Lindenberger, U., Rutishauser, U., & Garrett, D. D. (2023, February 23). Single-neuron spiking variability in hippocampus dynamically tracks sensory content during memory formation in humans. BioRxiv. https://doi.org/10.1101/2023.02.23.529684
Waschke, L., Kloosterman, N., Obleser, J., & Garrett, D. D. (2021). Behavior needs neural variability. Neuron, 109(5), 751–766. https://doi.org/10.1016/j.neuron.2021.01.023
Welford, A. T. (1965). Performance, biological mechanisms and age: A theoretical sketch. In A. T. Welford and J. E. Birren (Eds.) Behavior, aging and the nervous system (pp. 3–20). Charles C. Thomas.
Wells C., Ward L. M., Chua R., & Inglis J. T. (2005). Touch noise increases vibrotactile sensitivity in old and young. Psychological Science, 16(4), 313–320. https://doi.org/10.1111/j.0956-7976.2005.01533.x
Acronyms
ASL: Arterial spin labeling
BOLD: Blood oxygenation level dependent (BOLD) imaging
DA: Dopamine
DWI: Diffusion-weighted magnetic resonance imaging
EEG: Electroencephalography
fMRI: Functional magnetic resonance imaging
GABA: Gamma-aminobutyric acid
PET: Positron emission tomography
SPM: Statistical parametric mapping
VarTbx: Variability Toolbox