This is us: Fabio Bauer
How does our working memory work? Fabio Bauer, a PhD student in the ERC-funded Research Group Adaptive Memory and Decision Making, is investigating this question. In this interview, he explains how his machine-learning model can be used to simulate and test working memory processes. In our "This is us" format, colleagues share insights into their work and motivation.
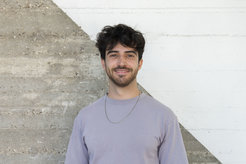
In the ERC-funded Research Group Adaptive Memory and Decision Making, you are working on machine learning models of working memory, among other things. What fascinates you about this topic?
Working memory fascinates me because, as temporary storage, it plays a central role in memory processes. It allows us to store and manipulate information for short periods of time. This is important for complex tasks such as problem-solving, language comprehension and decision making. Its capacity and efficiency significantly influence our ability to adapt to new situations and act flexibly. Understanding how working memory works and where its limits lie can help us better understand how the brain works and how we can handle information. One approach to better understanding is to use machine learning models to help us model and test the processes of working memory.
How does your machine learning model imitate the properties of human working memory?
The model we use can - like our human participants in our experiments - remember rotated images of 2D objects and rotate them back correctly when needed. The model consists of two main parts: a "visual system" and a "memory". The "visual system" greatly reduces the image and then reassembles it (Variational Autoencoder, or VAE), similar to how the human brain processes visual information. The "memory" (binding pool model) stores several reduced versions of the image as "tokens" but has a limited storage capacity. If it stores too many tokens, they will overlap, leading to errors. The model's memory therefore behaves in a similar way to working memory in humans, which also has limited capacity and shows overlap errors under load.
Can you explain your approach and results in more detail?
I use 2D images of clothes at different rotation angles as input data for my model. My VAE model has two separate areas: one for the shape/category of the clothes and one for their orientation. During training, the model alternately learns either the shape or the orientation, while the other part of the model is paused. This ensures that shape and orientation are represented separately. The information, such as “T-shirt” and rotation angle, is then stored in the model memory. The more information is stored, the more it overlaps. It will be exciting when we can compare this model data with the results of our experiments. Then we can answer whether the model represents the information in a similar format to the brain. So far, I don't have any results because I have just started my PhD and the project.
Why did you decide to use rotated 2D clothing items as stimuli for your model?
I use the open-source dataset “Fashion MNIST”, which is well-known in the machine learning community, as a basis. It contains 10 categories (pants, sweaters, etc.) with 7000 black and white 2D images of clothing items. Each image is then rotated to 16 different angles. Using rotated 2D clothing items as stimuli provides a clear and controlled way to study both shape and orientation processing. Clothing items have a variety of shapes that allow the model to learn complex patterns. By rotating the images, I can also test the model's ability to learn, remember and process abstract features such as orientation. In this way, the model can solve a similar task to that of human participants in our laboratory experiments, while at the same time investigating the effects and mechanisms of this working memory model.
When did you decide to become a scientist? How did you decide to study this subject?
Relatively late. After my bachelor's degree and a few years in a start-up, I realized that I lacked a sense of purpose in my work. Perhaps somewhat idealistically, I then applied for a master's degree in cognitive neuroscience. During my master's thesis, I really enjoyed computational and theoretical cognitive neuroscience, and that's how I came to do my PhD at the MPI.
What do you value about the Max Planck community?
What I particularly appreciate about the Max Planck community is the interdisciplinary and collaborative environment. The opportunity to work with leading experts from different disciplines and to have access to first-class resources and facilities is extremely valuable. The Max Planck Society promotes an environment in which innovative ideas and scientific excellence can flourish. In addition, the MPI offers numerous opportunities for exchange and further education.